Chen’s co-authored book on reduced-rank regression has been published by Springer.
Reinsel, G. C., Velu, R. P., and Chen, K. (2022) Multivariate Reduced-Rank Regression: Theory, Methods and Applications, 2nd Edition. Springer.
The book’s first edition has profoundly influenced Chen’s research since his graduate study at the University of Iowa under the supervision of Professor Kung-Sik Chan. Chen is honored and humbled to be a co-author in this new edition.
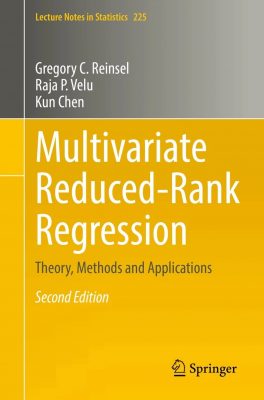
This book provides an account of multivariate reduced-rank regression, a tool of multivariate analysis that enjoys a broad array of applications. In addition to a historical review of the topic, its connection to other widely used statistical methods, such as multivariate analysis of variance (MANOVA), discriminant analysis, principal components, canonical correlation analysis, and errors-in-variables models, is also discussed.
This new edition incorporates Big Data methodology and its applications, as well as high-dimensional reduced-rank regression, generalized reduced-rank regression with complex data, and sparse and low-rank regression methods. Each chapter contains developments of basic theoretical results, as well as details on computational procedures, illustrated with numerical examples drawn from disciplines such as biochemistry, genetics, marketing, and finance.
This book is designed for advanced students, practitioners, and researchers, who may deal with moderate and high-dimensional multivariate data. Because regression is one of the most popular statistical methods, the multivariate regression analysis tools described should provide a natural way of looking at large (both cross-sectional and chronological) data sets. This book can be assigned in seminar-type courses taken by advanced graduate students in statistics, machine learning, econometrics, business, and engineering.